title: “ASSOCIATION BETWEEN OSTEOPOROSIS FRACTURES AND RACE IN UNITED STATES ADULTS USING THE 2017-2018 NATIONAL HEALTH AND NUTRITION EXAMINATION SURVEY (NHANES) DATA” output: github_document —
```{r setup} library(SASmarkdown)
## BACKGROUND
Osteoporosis is a common chronic disease of public health concern that results in fragility fractures affecting one in three women and one in five men over age 50 years. (IOF, 2020) This imposes a burden on the individuals and their caretakers result in morbidity and reduced quality of life.. Individuals who sustained osteoporotic fractures endure back pain, loss of height, loss of mobility and many other adverse effects on the quality of their lives. Over 1.5 million fractures per year occurs due to osteoporosis with 250,000 hip fracture, 500,000 hospitalizations, 800,000 emergency room visits, 2,600,000 physician visits, and 180,000 nursing home placements. This cost the Unites states $20 billion in 2015 (Rodan, 2000). Hip fractures are a leading cause of morbidity and loss of independence in the older population and a 1-year mortality of 20% (Johnell,2006). Osteoporotic fractures had resulted in an estimated loss of 5.8 million disability adjusted life years (DALYs) yearly (Johnell,2006).
These staggering cost on the health care system and the excruciating burden on the patient and the healthcare system need to be examined by public health professionals and medical personnel. Osteoporosis can be treated, and fractures prevented.
There are multiple factors that may explain the relationship between race/ethnicity and fracture risks. Race/ethnicity is a result of genetics which dictates the bone density, skeletal size, peak bone mass and body composition. Socio economic factors are as a result of disparities in social position in the society. (William, 2012) Those disparities influence the amount of sunlight exposure (related to education, income, neighborhood, crime rate, availability of parks, bicycle trails). The socioeconomic status also influences availability of primary prevention resources, smoking habits, alcohol consumption, comorbidities and proper diets. All these factors are interrelated as shown in the figure below:
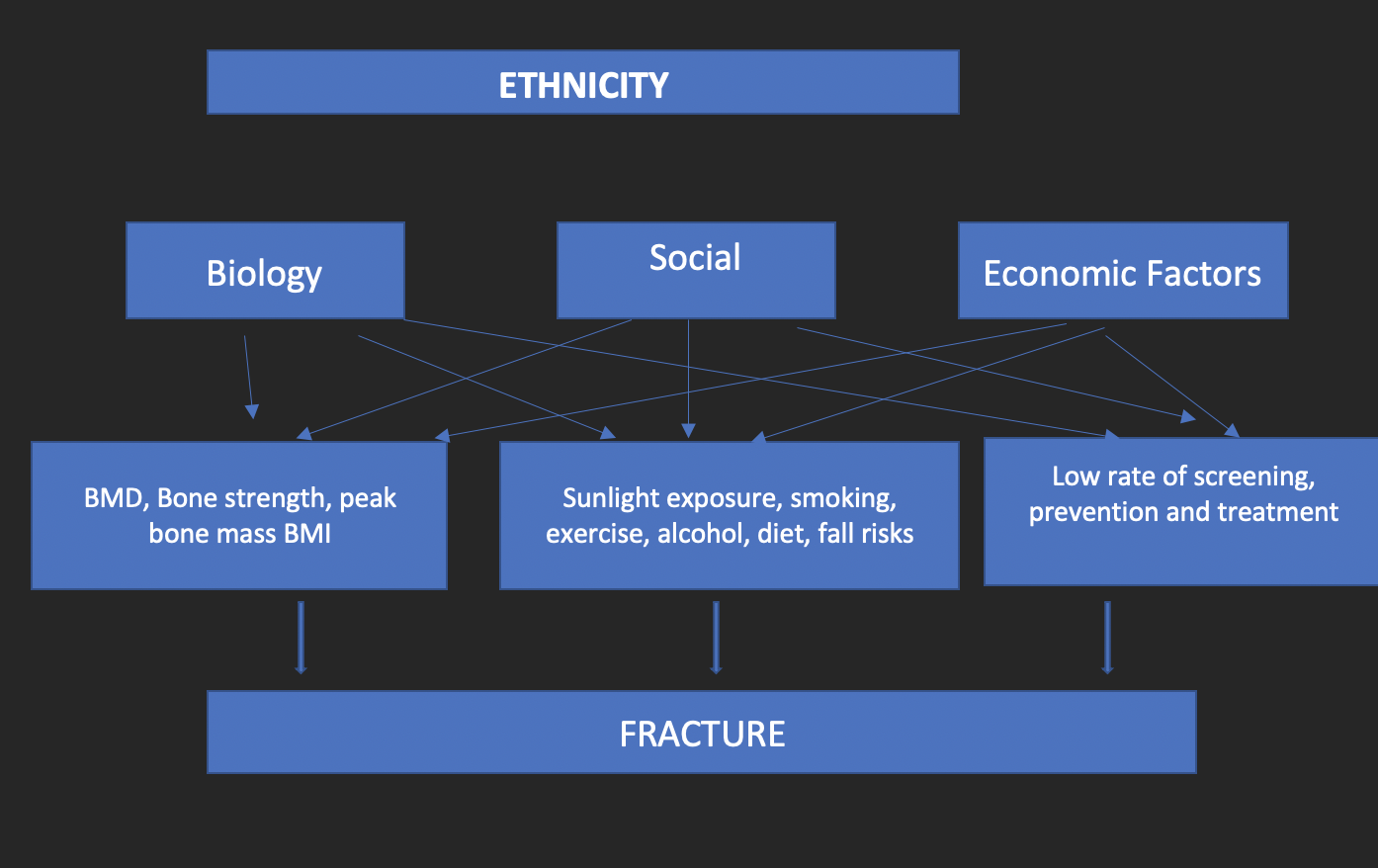
## AIM
- To determine the association between race and osteoporosis fracture
- To identify factors associated with osteoporosis fractures.
## METHODS
Data source is the National Health and Nutrition Examination Survey (NHANES) 2017- 2018, a nationally representative, cross-sectional survey designed to assess the health and nutritional status of adults and children across the United States. NHANES is a major program of the National Center for Health Statistics (NCHS) and is responsible for producing vital and health statistics for the Nation. (NCHS, 2017). The survey examines a national representative sample of individuals located in counties across the country.
The survey has examined a nationally representative sample of about 5,000 persons each year since
early 1960. These persons are in counties across the country, 15 of which are visited each year.
The NHANES interview includes demographic, socioeconomic, dietary, and health-related
questions. The examination component consists of medical, dental, and physiological measurements, as well as laboratory tests administered by highly trained medical personnel. This study uses data from the National Health and Nutrition Examination Survey, 2017-2018
(NHANES 2017-2018), collected between January 2017 and December 2018.
## Predictors
Fractures is the response variable.
The predictors include baseline characteristics, clinical risk factors and laboratory findings.
Gender, Age, Race, Marital status, Education, Calcium, Diabetes, Arthritis, Stroke, BMI,Alcohol intake, osteoporosis, prednisolone,family history of osteoporosis, smoking, smokingfrequency, T-Score of femur neck, femur neck bone mineral density, vigorous and moderate physical activity
## Data Preprocessing and Statistical Methods:
Analysis was conducted using RStudio Version 1.3.1073 and SAS studio. Data cleaning and preprocessing was done on RStudio while the data analysis was don on SASstudio.
The variables needed from NHANES 2017-2018 was selected, merged, and formatted
(Demographics, diet, smoking, alcohol, diabetes, fractures, osteoporosis, pregnancy, BMI, femoral bone mineral density, spine bone mineral density, calcium, and vitamin d levels, physical activity). This resulted in a total of 9253 individuals with 33 predictors.
The analysis was limited to individuals aged ≥50 years who were both interviewed and examined (3069 participants).
Subjects with missing data on bone mineral density (BMD) and fractures was excluded which makes the sample size to be 2286 with 28 predictors.
Variables with missing values of more than 40%: vit D- 52%, alcohol- 42%, smoking frequency- 54%, T-score of spine-49%, Spine Bone Mineral Density -49%, hormone replacement therapy-
55%. Poverty-income-ratio with missing value of 13% was also removed. One observation missing more than 2 features was removed as well. These leaves us with a data set of 2284 observations and 20 predictors. Each of the features had missing value of less than 5%. The sum of the missing values was 423 (18.5%) with a mean of 0.00925%
## Statistical Methods
Univariate analysis was conducted to describe the sample and estimate the prevalence of the racial groups and the prevalence of osteoporotic fractures. Descriptive statistics was calculated as percentages to describe the characteristics of the sample. Bivariate analysis was conducted thereafter to evaluate the relationship between race/ethnicity and osteoporotic fractures, tested using χ2 tests for proportions as appropriate.
Significant variables from the bivariate analysis were included in the stratified analysis (race, osteoporosis, smoking, alcohol intake, gender, T-score of femur neck, family history and age). Single factor stratification analysis to assess to for effect modification on the multiplicative and additive scale and for confounding using the Breslow- Day test of homogeneity, the stratum specific odds ratio and adjusted odds ratio ratios. Thereafter, multiple factors stratified analysis by smoking and gender was conducted to assess for effect modification 2 factors and for joint confounding.
I tested differences by gender, smoking and age in the association between race/ethnicity and osteoporotic fractures for significance by including first-order interaction terms (e.g., race*gender race*smoking race*age) in linear regression models. The interactions testing gender, smoking or age differences in the relationship between race/ethnicity and osteoporotic fractures were not significant at P < 0.05, so I opted not to include the interaction terms in the final models. Linear regression analysis was used when appropriate to assess the potential effect of race/ethnicity and osteoporotic fractures, after adjusting for race/ethnicity, gender, age and smoking, family history of osteoporosis, and osteoporosis diagnosis. Odds ratio is estimated based on logit- binomial distribution in the multivariable analysis. Descriptive and regression analyses will be conducted using SAS while all other statistical analyses will be performed with R software, and p < 0.05 is considered statistically significant.
```{sas}
FILENAME REFFILE '/home/u42976927/FinalProject.csv';
PROC IMPORT DATAFILE=REFFILE
DBMS=CSV
OUT=WORK.dat
replace;
GETNAMES=YES;
RUN;
data dat (drop=VAR1 femurneckBMD) ;
set WORK.dat;
run;
/* column list extracted */
proc contents data=dat out=column_nm varnum;
run;
proc print data= dat;
run;
-------------------------------------------------------------
/* Format the variables */
proc format;
value frac
1 = ' yes'
0 = 'no'
;
value $race
'Non-Hispanic White' =' White, NH'
'Non-Hispanic Blacks'=' Black, NH'
'Non-Hispanic Asian'= 'Asian, NH'
'Hispanic'= ' Hispanic'
'Other Race'= ' Other Race'
;
value $gender
'Male' = ' Male'
'Female' = 'Female'
;
value $Marital
'Married' = ' Married'
'Never Married' = ' Never Married'
'Previously Married'=' Previously Married'
'NA' = 'Missing'
;
value $calc
'high ca' = 'Missing'
'normal ca' = ' normal ca'
'low ca'=' low ca'
'NA' = 'Missing'
;
value $diab
'Yes' = ' Yes'
'No' = ' No'
'NA' = 'Missing'
;
value $arth
'Yes' = ' Yes'
'No' = ' No'
'NA' = 'Missing'
;
value $strok
'Yes' = ' Yes'
'No' = ' No'
'NA' = 'Missing'
;
value $bmi
'underweight' = ' underweight'
'normalweight' = ' normalweight'
'overweight' = ' overweight'
'obese' = ' obese'
'NA' = 'Missing'
;
value $alq
'Yes' = ' Yes'
'No' = ' No'
'NA' = 'Missing'
;
value $osteo
'Yes' = ' Yes'
'No' = ' No'
'NA' = 'Missing'
;
value $pred
'Yes' = ' Yes'
'No' = ' No'
'NA' = 'Missing'
;
value $fmhx
'Yes' = ' Yes'
'No' = ' No'
'NA' = 'Missing'
;
value $smk
'Yes' = ' Yes'
'No' = 'No'
;
value $edu
'noHS' = ' noHS'
'some/completedHS' = ' some/completedHS'
'highereducation' = ' highereducation'
'NA' = 'Missing'
;
value $Tscorefemurneck
'low bone mass' = ' low bone mass'
'normal' = 'normal'
'osteo' = ' osteo'
;
value $vig
'Vigorous' = ' Yes'
'NoVigorous' = ' No'
;
value $mod
'Moderate' = ' Yes'
'NoModerate' = ' No'
;
run;
*Univariate analysis Table 1*;
proc freq data=dat order= formatted;
format frac frac. calc $calc. ;
tables calc*frac;
run;
*Bivariate Table 2*;
proc freq data=dat order=formatted;
format frac frac. Gender $gender. race $race. Marital $Marital. calc $calc.
diab $diab. arth $arth. strok $strok. bmi $bmi. alq $alq. osteo $osteo. pred $pred.
fmhx $fmhx. smk $smk. edu $edu. vig $vig. mod $mod.;
tables mod*frac /cmh ;
run;
proc genmod data=dat order=formatted ;
format frac frac. Gender $gender. race $race. Marital $Marital. calc $calc.
diab $diab. arth $arth. strok $strok. bmi $bmi. alq $alq. osteo $osteo. pred $pred.
fmhx $fmhx. smk $smk. edu $edu.vig $vig. mod $mod. Tscorefemurneck $Tscorefemurneck.;
class race (ref='White, NH') osteo(ref='No') smk(ref='No') calc(ref='normal ca') alq(ref='No')
Gender(ref='Female')strok(ref='No') pred(ref='No') bmi(ref='normalweight') arth (ref= 'No')
Marital (ref='Married') edu(ref='highereducation') diab (ref='No')Tscorefemurneck(ref='normal')
fmhx(ref='No') age (ref='50-59')/param=ref;
model frac= bmi / dist=bin;
estimate "RR White" bmi 1 0 0 0 /exp;
estimate "RR Hispanic" bmi 0 1 0 0 /exp;
estimate "RR Black" bmi 0 0 1 0 /exp;
estimate "RR Black" bmi 0 0 0 1 /exp;
proc freq data=dat order=formatted;
format frac frac. Gender $gender. race $race. Marital $Marital. calc $calc.
diab $diab. arth $arth. strok $strok. bmi $bmi. alq $alq. osteo $osteo. pred $pred.
fmhx $fmhx. smk $smk. edu $edu. vig $vig. mod $mod.;
tables Gender age race Marital calc diab arth strok
bmi alq osteo pred fmhx smk edu Tscorefemurneck vig mod ;
run;
/*General association chi-square test*/;
proc freq data=dat order=formatted;
format frac frac. Gender $gender. race $race. Marital $Marital. calc $calc.
diab $diab. arth $arth. strok $strok. bmi $bmi. alq $alq. osteo $osteo. pred $pred.
fmhx $fmhx. smk $smk. edu $edu.vig $vig. mod $mod.;
tables mod*frac/chisq;
run;
*Test of trend*;
proc freq data=dat order=formatted;
format frac frac. ;
tables Tscorefemurneck*frac/trend norow nocol nopercent scores=table;
run;
*Bivariate, crude rr*;
*using black as reference*;
proc freq data=dat order= formatted;
format frac frac. race $race. ;
tables race*frac/ cmh ;
where race in ('Non-Hispanic White','Non-Hispanic Blacks');
run;
*using whites as reference*;
proc freq data=dat order= formatted;
format frac frac. ;
tables race*frac/ cmh;
where race in ('Non-Hispanic White','Non-Hispanic Blacks');
run;
*/Table 3 stratified analysis with breslow day test/*;
proc contents data=dat;
run;
data datt;
set dat;
if race = 'Non-Hispanic Blacks' then race = 'poc';
if race = 'Hispanic' then race = 'poc';
if race = 'Non-Hispanic White' then race = 'white';
run;
proc freq data=datt;
tables race*frac;
run;
#*bivariate poc vs whites*;#
proc freq data=datt order= formatted;
format frac frac. ;
tables race*frac/ cmh;
where race in ('poc','white');
run;
proc freq data= datt order=formatted;
format frac frac. Gender $gender. Marital $Marital. calc $calc.
diab $diab. arth $arth. strok $strok. bmi $bmi. alq $alq. osteo $osteo. pred $pred.
fmhx $fmhx. smk $smk. edu $edu.vig $vig. mod $mod. Tscorefemurneck $Tscorefemurneck.;
table fmhx*race*frac/OR cmh ;
where race in ('poc','white');
run;
*Final Table 4*;
proc genmod data=datt order=formatted ;
format frac frac. Gender $gender. Marital $Marital. calc $calc.
diab $diab. arth $arth. strok $strok. bmi $bmi. alq $alq. osteo $osteo. pred $pred.
fmhx $fmhx. smk $smk. edu $edu.vig $vig. mod $mod. Tscorefemurneck $Tscorefemurneck.;
class race (ref='white') osteo(ref='No') smk(ref='No') calc(ref='normal ca') alq(ref='No')
Gender(ref='Female') Tscorefemurneck(ref='normal') fmhx(ref='No') age (ref='50-59')/param=ref;
model frac= Race Gender age smk fmhx alq Tscorefemurneck / link=logit dist=bin ;
where race in ('poc','white');
estimate "POC" race 1 /exp;
estimate "male" Gender 1 /exp;
estimate "60-69" age 1 0 /exp;
estimate ">70" age 0 1 /exp;
estimate "smoking" smk 1 /exp;
estimate "Fam Hx" fmhx 1 0 /exp;
estimate "Alc" alq 1 0 /exp;
estimate "osteo" Tscorefemurneck 1 0 /exp;
estimate "lbm" Tscorefemurneck 0 1/exp;
run;
Table 1: Characteristics of the study population, National Health and Nutrition Examination Survey, 2017–2018.
There are 52% male and 48% females with 37% being between 50 to 59 years and 28% above 69 years. 37% are whites; NH, 22% Hispanics; 23% black NH and 14% are Asians. Majority of the study population were married (61%) and attained higher education (54%). More than half of the subjects had no comorbidities. 23% had diabetes, 45% had rheumatoid arthritis, 7% had stroke and 11% had osteoporosis. The calcium levels of 94% of the subjects was normal and 31% were obese. According to the family and social history, 86% consumed alcohol, 46% smoked cigarette, 13% had family history of osteoporosis and 38% performed moderate exercise. 12% had femoral neck T-score within the osteoporosis range and 7% has a history of prednisolone intake.
Table 2: Bivariate analysis of factors associated with fractures National Health and Nutrition Examination Survey, 2017–2018.
According to the bivariate analysis in Table 2; The predictors with statistically significant p- values of <0.05 includes race, calcium, Rheumatoid arthritis, stroke, alcohol intake, osteoporosis, prednisolone intake, family history, smoking history, and femoral neck T- score. Factors associated with an increased likelihood of fractures includes low calcium levels, rheumatoid arthritis, stroke, alcohol intake, prednisolone intake, family history, smoking and low bone mass. Hispanics and Blacks have a decreased likelihood of fractures.
Race/ethnicity is statistically significantly associated with having osteoporotic fractures (p<0.0001), with White, non-Hispanic seniors having the highest prevalence of 20% compared to 9% among black non-Hispanic, 11% among Hispanic, and 19% among Asian non-Hispanics. Fig 2 Blacks have 59% lower odds of fractures compared to whites, Hispanics have 50% lower odds of fractures compares to white, Asians have 75% lower odds of fractures compared to whites.
Those with stroke have a prevalence of 20% compared to 13% in those without stroke. Those with history of stroke have 1.7 times more odds of fractures compare to those with no history of stroke.
15% of those who consume alcohol have osteoporotic fractures compared to 5% in those who do not consume alcohol. Those who consume alcohol have 3 times more odds of fractures compare to those without a history of alcohol consumption.
81% reported to having no family history of osteoporosis. The prevalence of osteoporotic fractures in those with a family history is 16% compared to 13% in those without a family history of osteoporosis. Those with a family history of osteoporosis have 1.3 times more odds of fractures compare to those without a family history. This relationship is not statistically significant according to the confidence intervals 1.30(0.92, 1.83)
7.8% of those who smoke have osteoporotic fractures compared to 5.7% in those who do not smoke. Those who smoke have 1.7 times more odds of fractures compare to those who do not smoke cigarette.
According to the bivariate analysis, gender, age, marital status, diabetes, BMI, education, moderate and vigorous activity levels are not statistically significantly associated with having osteoporotic fractures. (p- values is not <0.05)
We then performed multivariate analyses to adjust the POR for factors that may confound the association between race/ethnicity and fractures.
Table 3: Stratified Analysis for the Association osteoporotic fracture and Race/Ethnicity, National Health and Nutrition Examination Survey, 2017–2018
From table 3, the association between non-Hispanic black versus white race and osteoporotic fractures remains strong after adjusting for each of the variables with a significant p<.0001 for all the variables. For the stratified analysis for the Association fracture and race/ethnicity, persons of color which comprised of blacks non-Hispanic, and Hispanics was compared to whites non-Hispanics - the reference group. People of color have 55% lower odds of fractures compared to whites non-Hispanics. The odds of osteoporotic fractures between persons of color and white seniors are higher among males than among females; in those 60-69 year in age; in smokers than in nonsmokers; in non-alcohol drinkers than in alcohol drinkers; in those with a no family history; and in those with osteoporosis according to the femur T-score.
Gender is a potential effect modifier. For men, the association between race and fractures is greater than it is for women. Using the 10% confounding change rule (CrudeRR-AdjustedRR/AdjustedRR) the covariates do not confound the relationship between race/ethnicity and osteoporotic fractures
Table 4 Adjusted Regression Models for the Association between osteoporotic fracture and Race/Ethnicity, National Health and Nutrition Examination Survey, 2017–2018
According to table 4; the association between race and osteoporotic fractures remains significant even when adjusted for age, gender, smoking in both model 1; and age, gender, smoking, family history of osteoporosis, alcohol intake and femoral T-score in model 2. People of color have a lower odd than whites by 55% in model 1 and 49% in model 2 which is consistent with the earlier results. Smoking, alcohol intake and femoral T- score are important predictors of osteoporotic fractures in the fully adjusted model and the final model. Interaction models were not included in the models because their effects were not significant and there were also no confounders.
This study using a national representative sample of the US population to investigate the osteoporotic fracture relationships with race/ethnicity. This study highlighted the prevalence of osteoporotic fractures among different racial and ethnic groups and also outlined the factors associated with increased fracture risks. There is consistent reduction in the fracture risks for African Americans. Whites, non-Hispanics have the highest rates followed by Hispanics. (Table 2) After adjusting for gender , which was an effect modifier, people of color which comprised of blacks non-Hispanic, and Hispanics had 55% lower odds of fractures compared to whites non-Hispanics. For the stratified analysis (Table 3) , males, cigarette smokers, non-alcohol drinkers, those with no family history are associated with an increased risk of osteoporotic fractures.
Further regression analysis where models were compares revealed that, in models adjusting for race/ethnicity, gender, age and smoking, family history of osteoporosis, alcohol intake and femoral T-score, the relationship between race/ethnicity and fracture risks remains the same. Factors associated with increased risk of fractures in the full model includes smoking, alcohol, and femoral T-score <-2.5.
This study is the only recent study that compared people of color (black non-Hispanics and Hispanics) to whites non-Hispanics. Other studies found were old studies and most compared whites with blacks. A few studies have used recent NHANE data to examine racial differences with osteoporotic fractures. This cohort study showed increased fracture risk in whites with age, and weight being effect modifiers (Barrett-Connor, 2005) This study using date from the Women’s Health Initiative found predictors for fracture for blacks to be at least high school education, positive fracture history, and more than two falls. (Cauley, 2007). My study has similar results with people of color having about 50% lower the rate of fractures than whites non-Hispanics. BMD is consistently associated with increased fracture risks (William, 2012; Braucer, 2009; Barrett-Connor, 2005), my study found an increased risk of osteoporotic fractures with abnormal T-score range of <-2.5 however the association was not significant after bivariate analysis (Table 3), significant results were found in the regression analysis using the full model..
The National Osteoporosis Foundation outlines the ethnic and racial disparities among the older Americans affecting mortality, morbidity and overall health cost. (NOF, 2021) Not much is done for those fracturing from osteoporosis on a national level. Although African Americans have a lower rate of fractures, according to our results, black Americans have a 54% lower rate of osteoporotic fracture, they have the highest hospitalization, low screening rates and mortality rates. (NOF, 2021) It is important to examine data and conduct more research to be able to adequately deal with this disparity through improved access to fracture after care in the POC communities, health education program and introduction of community based primary care utilizing members of the community. Provision of culturally sensitive information and care is needed to encourage access to care. Further research and follow up should be done in the area of screening, awareness and provision of policies and laws targeted to early diagnosis and screening, treatment, rehabilitation and overall improvement of bone health among minority population.
In conclusion, this study investigated the relationship between race/ethnicity and osteoporotic fracture and found that people of color have about 50% lower rate of osteoporotic fracture than black non-Hispanics whites. Smoking cigarettes, alcohol intake, and femoral T-score <-2.5 are associated with an increased risk of osteoporotic fractures. Despite people of color having the lowest risk of osteoporotic fractures, there have the worst out comes. Black women are less knowledgeable about osteoporosis than white women (Wright, 2017), they have lower screening and less likely to be treated (Curtis, 2009). The have the highest hospitalization and mortality rates (NOF, 2021). More needs to be done in primary, secondary and tertiary prevention of osteoporotic fractures in African American women
Barrett-Connor, E., Siris, E. S., Wehren, L. E., Miller, P. D., Abbott, T. A., Berger, M. L., Santora, A. C., & Sherwood, L. M. (2005). Osteoporosis and fracture risk in women of different ethnic groups. Journal of bone and mineral research: the official journal of the American Society for Bone and Mineral Research, 20(2), 185–194. https://doi.org/10.1359/JBMR.041007
Brauer CA, Coca-Perraillon M, Cutler DM, Rosen AB. Incidence and mortality of hip fractures in the United States. JAMA. 2009;302:1573–1579. doi: 10.1001/jama.2009.1462. [PMC free article] [PubMed] [CrossRef] [Google Scholar]
Cauley, J. A., Wu, L., Wampler, N. S., Barnhart, J. M., Allison, M., Chen, Z., Jackson, R., & Robbins, J. (2007). Clinical risk factors for fractures in multi-ethnic women: the Women’s Health Initiative. Journal of bone and mineral research : the official journal of the American Society for Bone and Mineral Research, 22(11), 1816–1826. https://doi.org/10.1359/jbmr.070713
Curtis JR, McClure LA, Delzell E, et al. Population-based fracture risk assessment and osteoporosis treatment disparities by race and gender. J Gen Intern Med. 2009;24(8):956-962. doi:10.1007/s11606-009-1031-8
Harvey NCW, McCloskey EV et al. 2016 IOF World Osteoporosis Day Report
Johnell O, Kanis JA (2006) An estimate of the worldwide prevalence and disability associated with osteoporotic fractures. Osteoporos Int 17(12):1726–1733
Kanis JA on behalf of the World Health Organization Scientific Group. Assessment of osteoporosis at the primary health care level. Technical report. Sheffield, UK: World Health Organization Collaborating Centre for Metabolic Bone Diseases, University of Sheffield. 2007.